Mamba: A New Frontier in Sequence Modeling
Mamba is an innovative model revolutionizing sequence modeling, surpassing traditional Transformer architectures in efficiency and performance. Its design specifically addresses the limitations of Transformers, especially in handling longer sequences, making it a significant advancement in the field of machine learning.
DIGITALEVOLUTIONAIAI MODELS
Yasir Bucha
1/27/20241 min read
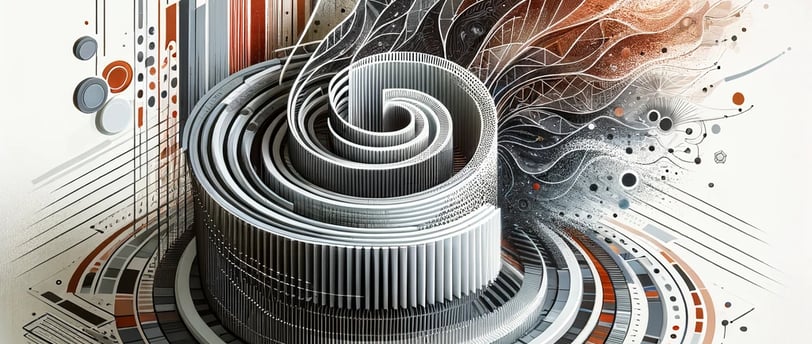
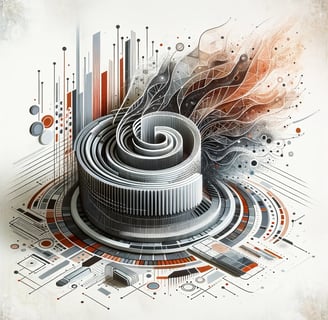
Mamba, developed by Albert Gu and Tri Dao, stands out in its ability to process complex sequences across various fields like language processing, genomics, and audio analysis. It utilizes a linear-time sequence modeling approach with selective state spaces, ensuring superior performance. This selective state space method allows for faster inference and better scalability with sequence length, greatly improving throughput over traditional Transformer models.
One of the key advantages of Mamba is its unique architecture that simplifies the modeling process while maintaining high efficiency. Unlike Transformers, which struggle with long sequences due to their complex attention mechanisms, Mamba employs a selective approach in state space models. This not only enables more efficient processing but also allows for linear scaling with sequence length. Its hardware-friendly design, inspired by FlashAttention, allows Mamba to outperform many existing models, marking it as a noteworthy advancement in machine learning.
Reflecting on Mamba's impact, its introduction is a testament to the continual evolution of machine learning models. Mamba's approach to sequence modeling, prioritizing efficiency and scalability, could redefine how we tackle complex data analysis tasks. Its ability to handle diverse data types and large-scale applications showcases the dynamic nature of AI development, potentially opening new doors in various scientific and technological fields.
#AIRevolution #MachineLearningInnovation #SequenceModeling
NovoSphere
Creating Convenience Through Innovation
Copyrights © 2023 - NovoSphere | All rights Reserved
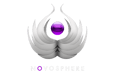
